Set the Floor for Success: Testing and Experimentation for Effective Custom Evaluations for AI
Set the Floor for Success: Testing and Experimentation for Effective Custom Evaluations for AI
Set the Floor for Success: Testing and Experimentation for Effective Custom Evaluations for AI
At Ownlayer, we empower organizations to deliver exceptional AI experiences at scale. Our collaboration with diverse clients has given us unique insights into the strategies that drive high-performing AI teams. In this second blog post of our 3-part playbook series, we focus on testing and experimentation, highlighting how to build effective custom evaluations to ensure your AI models meet specific use case requirements and deliver consistent value.
Building Effective Custom Evaluations
In our previous blog on in-production monitoring, we established the importance of monitoring AI quality through custom evaluators. The level of customization often requires a fusion of deep product insight and sector-specific expertise, knowledge that often extends far beyond the scope of typical development teams. How exactly do you build effective custom evaluations? Here, we break down the process of some of the best practices we’ve seen:
Engage Your Stakeholders
Involve your stakeholders early. They may be product experts, customer success leads, legal and compliance teams, or other stakeholders who are responsible for delivering exceptional UX. Some of the best Legal AI products we’ve seen have over 10 legal experts manually reviewing responses before automating through Ownlayer.
Provide ways for them to easily create, review, and provide feedback on the evaluators being implemented, ideally in natural language.
Break Down Your Potential Use Cases and Themes
Map out how you expect users to interact with the product.
Identify use cases and group them into themes to provide a clear focus for evaluations.
Prioritize among the use cases for future cost and alert optimization.
Create Detailed Criteria for Each Use Case
For each use case, define a detailed list of criteria that defines what "good" performance looks like. For example, a good inbound leads qualification AI needs to have clear follow-up steps including emails, follow-up Zoom setup, and/or connections to other team members. If you’re using AI to extract key information from a document, such as income proof, define clearly what constitutes an efficient proof.
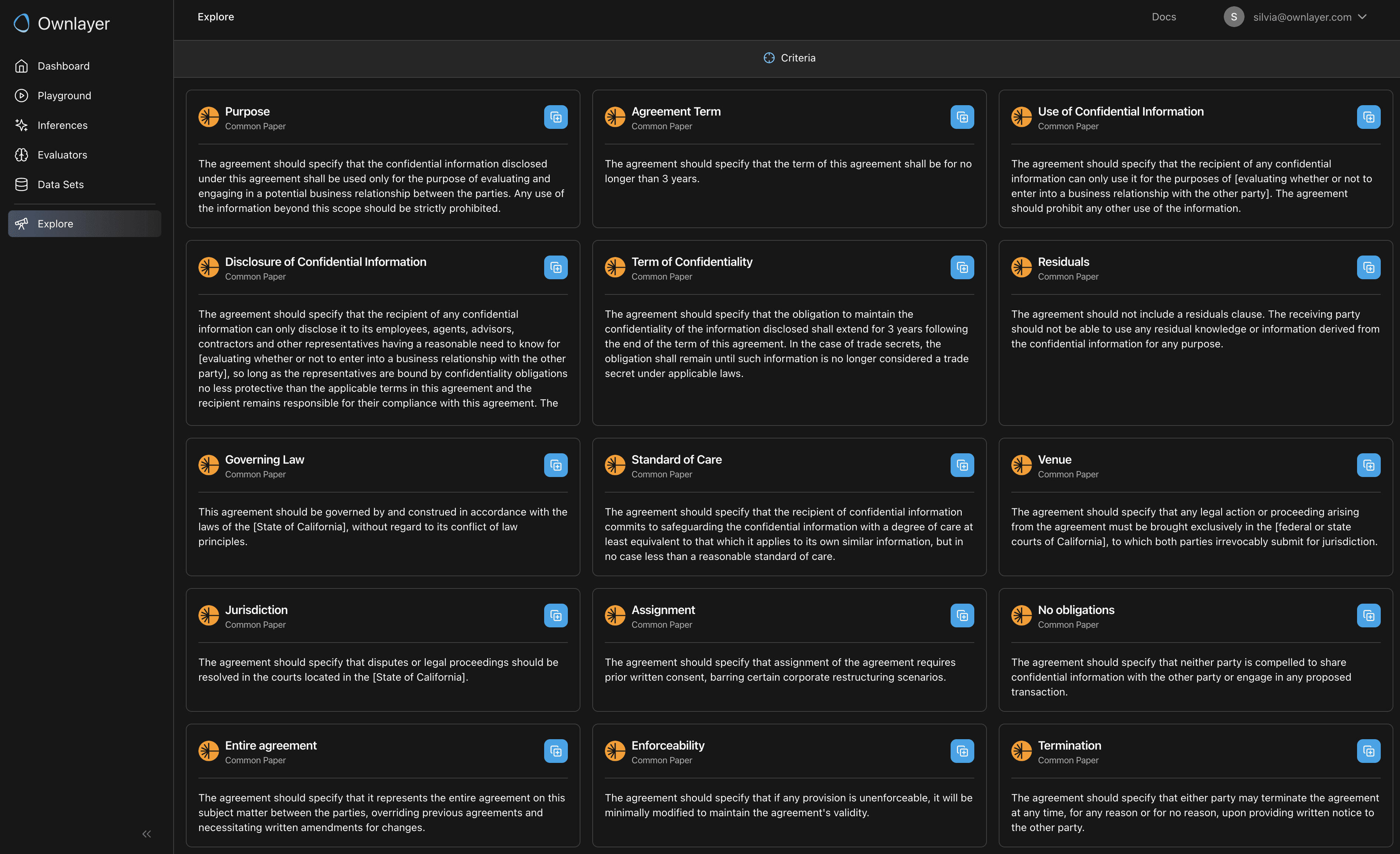
Test to Find the Most Effective Evaluators for Each Criterion
Break down criteria into small, specific components to allow for more accurate and clear responses.
Choose different LLM configurations to power your evaluators for cost-effective evaluations.
Experiment and test each criterion using different evaluators, ideally on a sample of your production dataset.
Ongoing Testing and Experimentation
While your AI feature is in production, you should expect to continuously refine and improve your evaluators. This process includes:
Curate data sets consisting of production logs that highlight corner cases.
Create new evaluators to capture additional corner cases.
Sunset legacy evaluators that are outdated or perform poorly.
Conclusion
Testing and experimentation are crucial for deploying AI features that meet specific use case requirements and deliver consistent value. By involving stakeholders, breaking down use cases, defining detailed criteria, testing with various evaluators, and building the experimentation into an ongoing flow, organizations can build effective custom evaluations. This structured approach ensures the AI system aligns with business goals and user expectations.
This is the second part of our 3-part playbook series. In the next blog, we'll dive into post-production analytics to complete your AI implementation strategy.
Ready to elevate your AI strategy? Find us at Ownlayer to learn how Ownlayer can help you implement effective pre-production testing and experimentation tailored to your specific needs.
At Ownlayer, we empower organizations to deliver exceptional AI experiences at scale. Our collaboration with diverse clients has given us unique insights into the strategies that drive high-performing AI teams. In this second blog post of our 3-part playbook series, we focus on testing and experimentation, highlighting how to build effective custom evaluations to ensure your AI models meet specific use case requirements and deliver consistent value.
Building Effective Custom Evaluations
In our previous blog on in-production monitoring, we established the importance of monitoring AI quality through custom evaluators. The level of customization often requires a fusion of deep product insight and sector-specific expertise, knowledge that often extends far beyond the scope of typical development teams. How exactly do you build effective custom evaluations? Here, we break down the process of some of the best practices we’ve seen:
Engage Your Stakeholders
Involve your stakeholders early. They may be product experts, customer success leads, legal and compliance teams, or other stakeholders who are responsible for delivering exceptional UX. Some of the best Legal AI products we’ve seen have over 10 legal experts manually reviewing responses before automating through Ownlayer.
Provide ways for them to easily create, review, and provide feedback on the evaluators being implemented, ideally in natural language.
Break Down Your Potential Use Cases and Themes
Map out how you expect users to interact with the product.
Identify use cases and group them into themes to provide a clear focus for evaluations.
Prioritize among the use cases for future cost and alert optimization.
Create Detailed Criteria for Each Use Case
For each use case, define a detailed list of criteria that defines what "good" performance looks like. For example, a good inbound leads qualification AI needs to have clear follow-up steps including emails, follow-up Zoom setup, and/or connections to other team members. If you’re using AI to extract key information from a document, such as income proof, define clearly what constitutes an efficient proof.
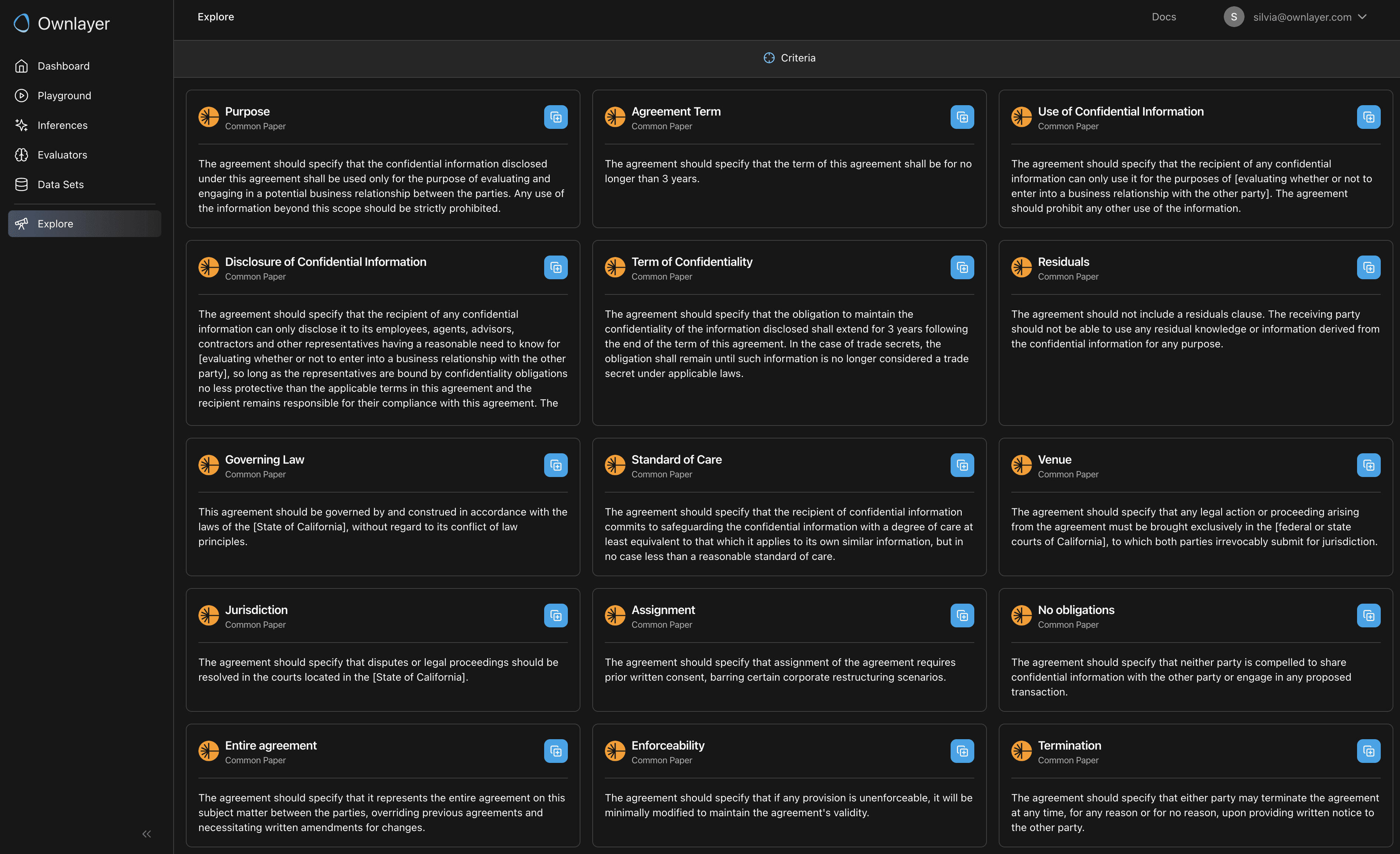
Test to Find the Most Effective Evaluators for Each Criterion
Break down criteria into small, specific components to allow for more accurate and clear responses.
Choose different LLM configurations to power your evaluators for cost-effective evaluations.
Experiment and test each criterion using different evaluators, ideally on a sample of your production dataset.
Ongoing Testing and Experimentation
While your AI feature is in production, you should expect to continuously refine and improve your evaluators. This process includes:
Curate data sets consisting of production logs that highlight corner cases.
Create new evaluators to capture additional corner cases.
Sunset legacy evaluators that are outdated or perform poorly.
Conclusion
Testing and experimentation are crucial for deploying AI features that meet specific use case requirements and deliver consistent value. By involving stakeholders, breaking down use cases, defining detailed criteria, testing with various evaluators, and building the experimentation into an ongoing flow, organizations can build effective custom evaluations. This structured approach ensures the AI system aligns with business goals and user expectations.
This is the second part of our 3-part playbook series. In the next blog, we'll dive into post-production analytics to complete your AI implementation strategy.
Ready to elevate your AI strategy? Find us at Ownlayer to learn how Ownlayer can help you implement effective pre-production testing and experimentation tailored to your specific needs.
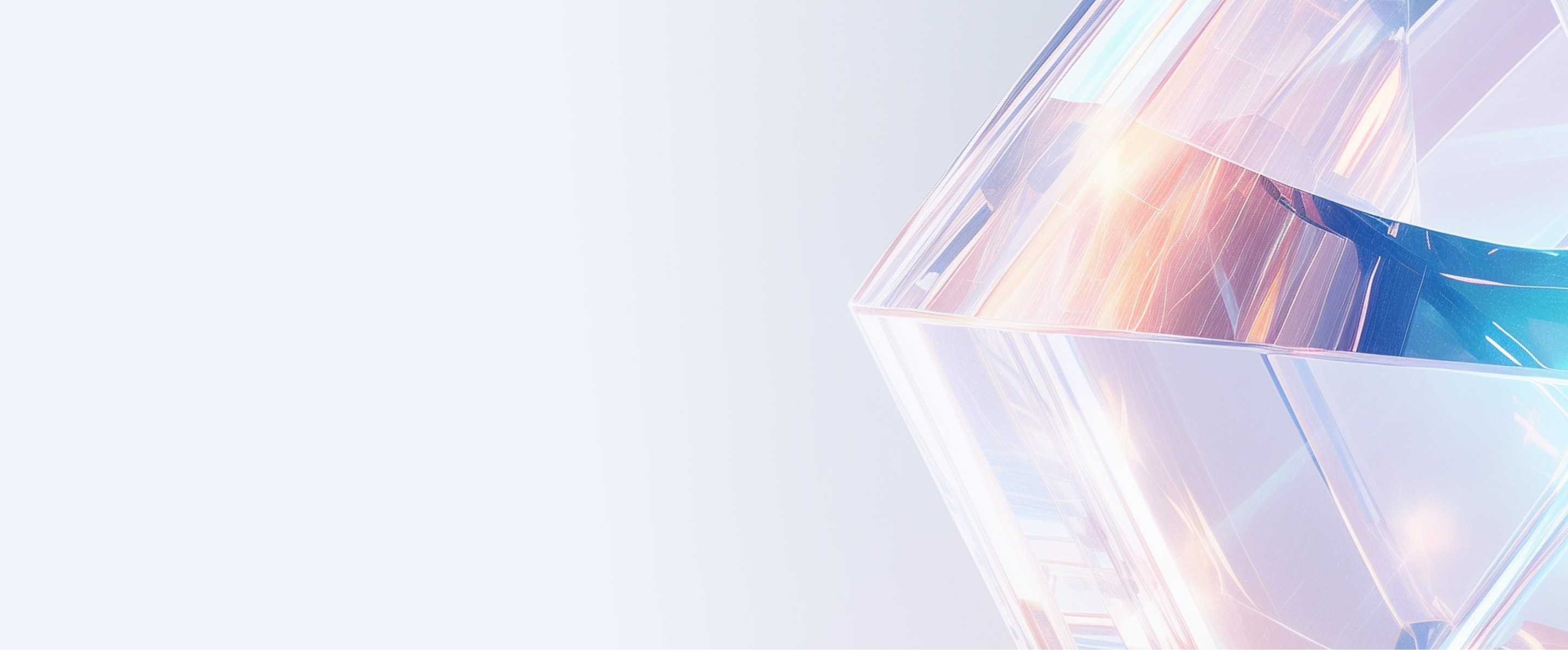
From Data to Decision, We Help You Deploy AI with Confidence
From Data to Decision, We Help You Deploy AI with Confidence
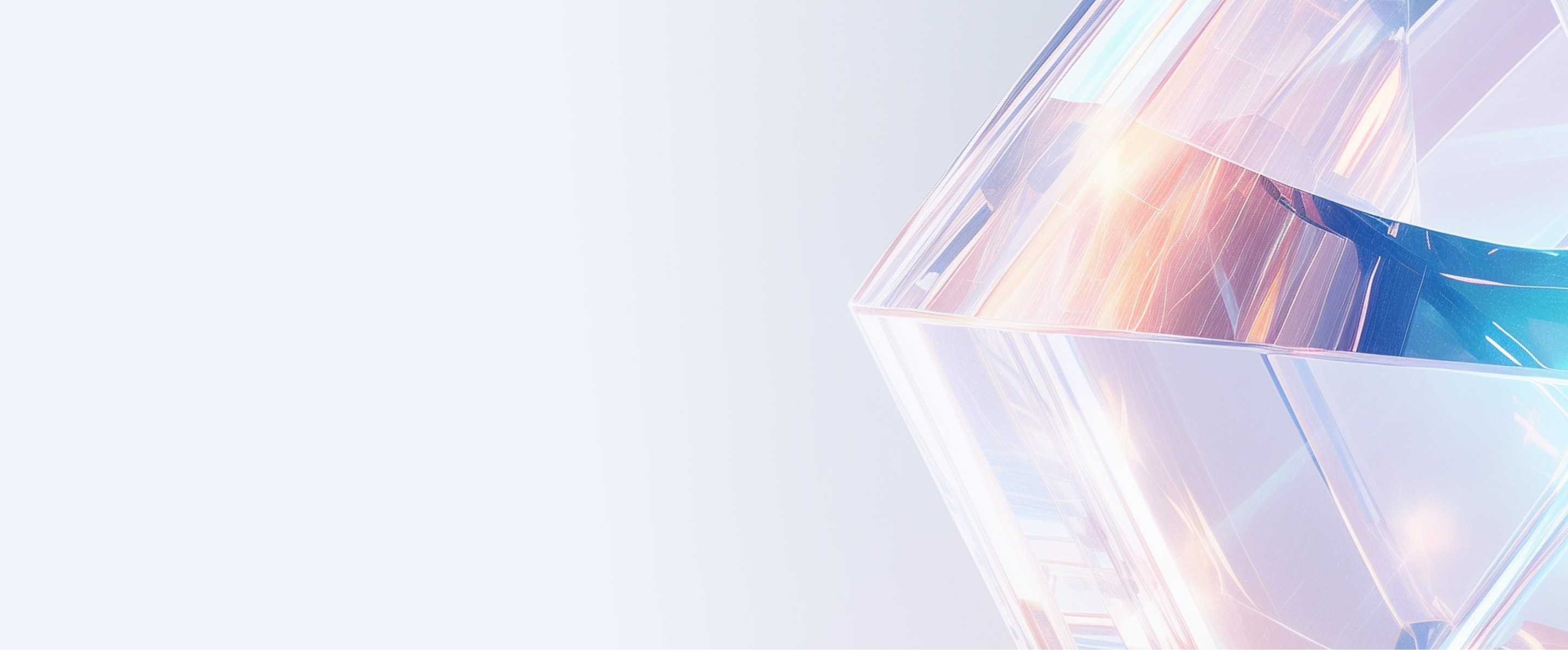
From Data to Decision, We Help You Deploy AI with Confidence
© Ownlayer, Inc 2025. All rights reserved.
© Ownlayer, Inc 2025. All rights reserved.
© Ownlayer, Inc 2025. All rights reserved.